비즈니스 인텔리전스 분석가
노동통계국에서 이 직업에 대한 구체적인 고용 데이터를 제공하지 않기 때문에, 우리는 Data Scientists에서 제공하는 데이터를 사용하고 있습니다.
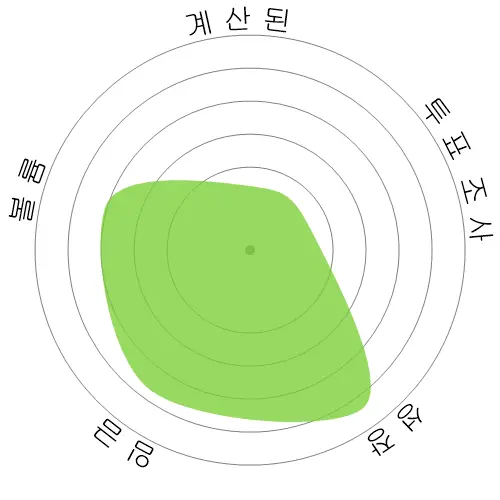



사람들이 또한 조회했습니다
계산된 자동화 위험
고위험 (61-80%): 이 범주에 속하는 직업은 현재 또는 가까운 미래의 기술을 사용하여 쉽게 자동화될 수 있는 많은 업무를 수행하므로 자동화로부터 중대한 위협을 받고 있습니다.
이 점수가 무엇인지, 그리고 어떻게 계산되는지에 대한 자세한 정보는 여기에 있습니다.
사용자 설문조사
우리의 방문자들은 이 직업이 자동화 될 가능성이 높다고 투표했습니다. 이 평가는 계산된 자동화 위험 수준에 의해 더욱 지지받고 있으며, 이는 자동화의 74% 확률을 추정합니다.
자동화의 위험성에 대해 어떻게 생각하십니까?
비즈니스 인텔리전스 분석가이 다음 20년 이내에 로봇이나 인공지능에 의해 대체될 가능성은 얼마나 됩니까?
감정
다음 그래프는 의미 있는 데이터를 제공할 수 있을 만큼 충분한 투표 수가 있을 때마다 포함됩니다. 이러한 시각적 표현은 시간 경과에 따른 사용자 투표 결과를 보여주며, 감정 추세에 대한 중요한 지표를 제공합니다.
시간별 감정 (연간)
성장
'Data Scientists' 직업 분야의 공석은 2033년까지 36.0% 증가할 것으로 예상됩니다.
업데이트된 예상치가 09-2024에 제출될 예정입니다..
임금
2023년에 'Data Scientists'의 중앙값 연간 급여는 $108,020이며, 시간당 $51입니다.
'Data Scientists'은 전국 중위임금인 $48,060보다 124.8% 더 높은 금액을 지불받았습니다.
볼륨
2023년 현재, 미국 내에서 'Data Scientists'로 고용된 사람들의 수는 192,710명이었습니다.
이는 전국의 고용 노동력 중 약 0.13%를 대표합니다.
다시 말해, 약 787명 중 1명이 'Data Scientists'로 고용되어 있습니다.
직무 설명
데이터 저장소를 쿼리하여 금융 및 시장 정보를 생성하고 주기적인 보고서를 작성합니다. 사용 가능한 정보 소스에서 데이터 패턴과 추세를 식별하는 방법을 고안합니다.
SOC Code: 15-2051.01
자원
당신의 지역에서 일자리를 검색하세요
새로운 커리어를 시작하려고 생각하거나 직장을 바꾸려는 계획이 있다면, 우리가 만든 편리한 채용 검색 도구를 사용해보세요. 이 도구를 통해 완벽한 새로운 역할을 찾을 수 있을지도 모릅니다.
댓글
Leave a comment
The bulk of my time as a BI analyst is spent on 2 things. The first is working with domain stakeholders without technical knowledge (marketers in my case). This can often be like herding cats. Lots of people will have lots of different opinions on what KPIs they want to track or how to track them. And often they don't really understand the data limitations of what we can and can't report on, so I need to be there to provide guidance. Much of my job is spent guiding these people along, often massaging their egos along the way, so that the wider group of people arrives at a consensus.
The second is ETL. Even with whole teams of data engineers and operations managers, data is very rarely centralised into a single and easy to understand model. I work with about half a dozen different types of data sources (from AWS to Google Sheets). Each of these have hundreds of different indexes and many of those indexes have hundreds of fields. A tiny fraction of these fields have any kind of documentation and so all you have to go on is the metadata and the name of the db managers who put it together. Actually tracking down the data you need requires getting really into the weeds and following up with multiple people to try to track down who actually knows where to find the data you're looking for. That's just nowhere near enough data for an AI to get a hold of the data it needs.
It is a field where people usually believe in other people rather than AI.
The ETL process is also a complicated, one which most AI is not nor ever may be able to handle, data needs to be cleaned and standardized before AI can take a crack at it, the "AI" and yes I have to put that in quotes does not understand the context of anything, it is a prediction model using gradient boosters that performs quite well under controlled circumstances, thrown into any critical thought role it starts to lose pace. Furthermore nobody who works in the AI space authoring models ands understands the inner workings of "AI" treats this as anything more than a highly sophisticated toy...maybe in another 10 years we can come back to this question and see if we should start to worry.
I spend half of my time maintaining, tweaking, and fixing automation jobs - these include dashboards, data sets, and database tables.
It requires a technical person who is also an expert in their business domain to translate business requirements into data or reports that others need.
이 직업에 대한 답글을 남겨주세요.