Analitycy ds. Inteligencji Biznesowej
Dane zatrudnienia nie są dostępne specjalnie dla tego zawodu z Biura Statystyki Pracy, więc korzystamy z danych z Data Scientists.
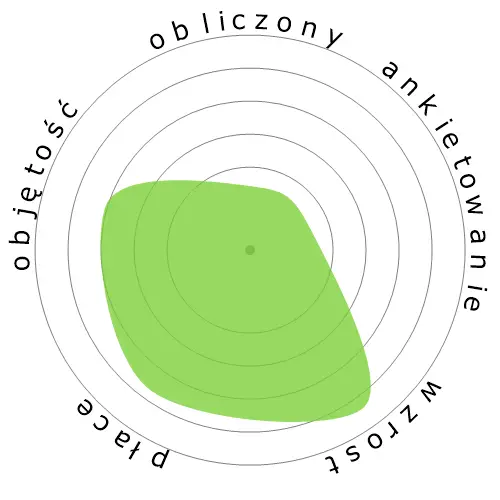



Chcesz ten podsumowanie na swojej stronie? Kod do umieszczenia:
Ryzyko automatyzacji
Wysokie ryzyko (61-80%): Zawody w tej kategorii stoją przed znaczącym zagrożeniem ze strony automatyzacji, ponieważ wiele z ich zadań może być łatwo zautomatyzowanych przy użyciu obecnych lub bliskich przyszłości technologii.
Więcej informacji na temat tego, czym jest ten wynik i jak jest obliczany, jest dostępne tutaj.
Ankieta użytkownika
Nasi goście głosowali, że nie są pewni, czy to zawód zostanie zautomatyzowany. Jednakże, poziom ryzyka automatyzacji, który wygenerowaliśmy, sugeruje znacznie większą szansę na automatyzację: 69% szans na automatyzację.
Jakie są Twoje zdanie na temat ryzyka automatyzacji?
Jakie jest prawdopodobieństwo, że Analitycy ds. Inteligencji Biznesowej zostanie zastąpione przez roboty lub sztuczną inteligencję w ciągu najbliższych 20 lat?
Nastroje
Następujące wykres(y) są dołączane tam, gdzie jest znaczna liczba głosów, które umożliwiają uzyskanie sensownych danych. Te wizualne reprezentacje pokazują wyniki ankiety użytkowników na przestrzeni czasu, dostarczając istotnych wskazówek dotyczących trendów nastrojów.
Nastroje w czasie (rocznie)
Wzrost
Liczba ofert pracy na stanowisku 'Data Scientists' ma wzrosnąć 35,8% do 2032
Zaktualizowane prognozy mają być dostępne 09-2023.
Płace
W 2022, mediana rocznej pensji dla 'Data Scientists' wynosiła 103 500 $, czyli 49 $ za godzinę.
'Data Scientists' otrzymali wynagrodzenie wyższe o 123,5% od średniej krajowej, która wynosiła 46 310 $
Objętość
Od 2022 roku zatrudnionych było 159 630 osób na stanowisku 'Data Scientists' w Stanach Zjednoczonych.
To oznacza około 0,11% zatrudnionej siły roboczej w całym kraju.
Inaczej mówiąc, około 1 na 926 osób jest zatrudnionych jako 'Data Scientists'.
Opis stanowiska pracy
Produkuj informacje finansowe i rynkowe, odpytując repozytoria danych i generując okresowe raporty. Opracuj metody identyfikacji wzorców i trendów danych w dostępnych źródłach informacji.
SOC Code: 15-2051.01
Zasoby
Jeśli myślisz o rozpoczęciu nowej kariery lub szukasz zmiany pracy, stworzyliśmy przydatne narzędzie do wyszukiwania pracy, które może Ci pomóc zdobyć tę idealną nową rolę.
Komentarze
The ETL process is also a complicated, one which most AI is not nor ever may be able to handle, data needs to be cleaned and standardized before AI can take a crack at it, the "AI" and yes I have to put that in quotes does not understand the context of anything, it is a prediction model using gradient boosters that performs quite well under controlled circumstances, thrown into any critical thought role it starts to lose pace. Furthermore nobody who works in the AI space authoring models ands understands the inner workings of "AI" treats this as anything more than a highly sophisticated toy...maybe in another 10 years we can come back to this question and see if we should start to worry.
I spend half of my time maintaining, tweaking, and fixing automation jobs - these include dashboards, data sets, and database tables.
It requires a technical person who is also an expert in their business domain to translate business requirements into data or reports that others need.
Zostaw odpowiedź na temat tego zawodu