Analisti di Business Intelligence
I dati sull'occupazione non sono disponibili specificamente per questa professione dal Bureau of Labor Statistics, quindi stiamo utilizzando i dati provenienti da Data Scientists.
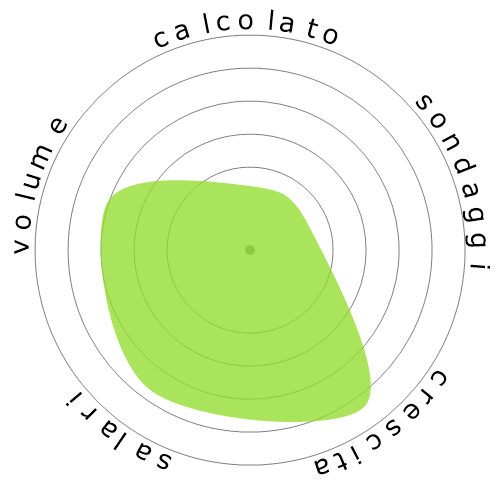



Le persone hanno anche visualizzato
Rischio di automazione calcolato
Rischio Alto (61-80%): Le professioni in questa categoria affrontano una minaccia significativa dall'automazione, poiché molte delle loro attività possono essere facilmente automatizzate utilizzando le tecnologie attuali o di prossima generazione.
Ulteriori informazioni su cosa sia questo punteggio e su come viene calcolato sono disponibili qui.
Sondaggio degli utenti
I nostri visitatori hanno votato che è probabile che questa professione sarà automatizzata. Questa valutazione è ulteriormente supportata dal livello di rischio di automazione calcolato, che stima una possibilità di automazione del 74%.
Cosa pensi sia il rischio dell'automazione?
Qual è la probabilità che Analisti di Business Intelligence venga sostituito da robot o intelligenza artificiale nei prossimi 20 anni?
Sentimento
Il seguente grafico è incluso ovunque ci sia una quantità sostanziale di voti per rendere i dati significativi. Queste rappresentazioni visive mostrano i risultati dei sondaggi degli utenti nel tempo, fornendo un'indicazione significativa delle tendenze di sentimento.
Sentimento nel tempo (annuale)
Crescita
Il numero di offerte di lavoro per 'Data Scientists' dovrebbe aumentare 36,0% entro il 2033
Le previsioni aggiornate sono previste per 09-2025.
Salari
Nel 2023, il salario annuo mediano per 'Data Scientists' era di 108.020 $, o 51 $ all'ora.
'Data Scientists' hanno ricevuto un salario 124,8% superiore al salario mediano nazionale, che si attestava a 48.060 $
Volume
A partire dal 2023 c'erano 192.710 persone impiegate come 'Data Scientists' negli Stati Uniti.
Questo rappresenta circa il 0,13% della forza lavoro impiegata in tutto il paese
In altre parole, circa 1 su 787 persone sono impiegate come 'Data Scientists'.
Descrizione del lavoro
Producete informazioni finanziarie e di mercato interrogando i repository di dati e generando rapporti periodici. Elaborate metodi per identificare schemi e tendenze nei dati disponibili dalle fonti di informazione.
SOC Code: 15-2051.01
Commenti
Leave a comment
The bulk of my time as a BI analyst is spent on 2 things. The first is working with domain stakeholders without technical knowledge (marketers in my case). This can often be like herding cats. Lots of people will have lots of different opinions on what KPIs they want to track or how to track them. And often they don't really understand the data limitations of what we can and can't report on, so I need to be there to provide guidance. Much of my job is spent guiding these people along, often massaging their egos along the way, so that the wider group of people arrives at a consensus.
The second is ETL. Even with whole teams of data engineers and operations managers, data is very rarely centralised into a single and easy to understand model. I work with about half a dozen different types of data sources (from AWS to Google Sheets). Each of these have hundreds of different indexes and many of those indexes have hundreds of fields. A tiny fraction of these fields have any kind of documentation and so all you have to go on is the metadata and the name of the db managers who put it together. Actually tracking down the data you need requires getting really into the weeds and following up with multiple people to try to track down who actually knows where to find the data you're looking for. That's just nowhere near enough data for an AI to get a hold of the data it needs.
It is a field where people usually believe in other people rather than AI.
The ETL process is also a complicated, one which most AI is not nor ever may be able to handle, data needs to be cleaned and standardized before AI can take a crack at it, the "AI" and yes I have to put that in quotes does not understand the context of anything, it is a prediction model using gradient boosters that performs quite well under controlled circumstances, thrown into any critical thought role it starts to lose pace. Furthermore nobody who works in the AI space authoring models ands understands the inner workings of "AI" treats this as anything more than a highly sophisticated toy...maybe in another 10 years we can come back to this question and see if we should start to worry.
I spend half of my time maintaining, tweaking, and fixing automation jobs - these include dashboards, data sets, and database tables.
It requires a technical person who is also an expert in their business domain to translate business requirements into data or reports that others need.
Lascia un commento su questa professione