Analistas de Inteligência de Negócios
Dados de emprego não estão disponíveis especificamente para esta ocupação no Bureau of Labor Statistics, então estamos usando os dados de Data Scientists.
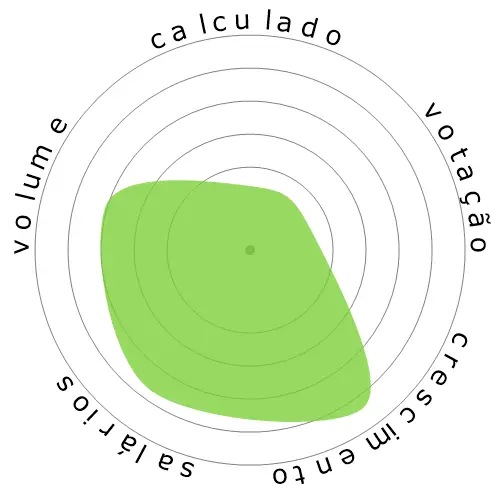



As pessoas também visualizaram
Risco de automação calculado
Alto Risco (61-80%): Empregos nesta categoria enfrentam uma ameaça significativa da automação, pois muitas de suas tarefas podem ser facilmente automatizadas usando tecnologias atuais ou de futuro próximo.
Mais informações sobre o que é essa pontuação e como ela é calculada estão disponíveis aqui.
Enquete do usuário
Nossos visitantes votaram que é provável que esta ocupação seja automatizada. Esta avaliação é ainda mais apoiada pelo nível de risco de automação calculado, que estima 74% de chance de automação.
O que você acha que é o risco da automação?
Qual é a probabilidade de que Analistas de Inteligência de Negócios seja substituído por robôs ou inteligência artificial nos próximos 20 anos?
Sentimento
O gráfico a seguir é incluído sempre que há uma quantidade substancial de votos para gerar dados significativos. Essas representações visuais mostram os resultados das enquetes dos usuários ao longo do tempo, fornecendo uma indicação importante das tendências de sentimento.
Sentimento ao longo do tempo (anualmente)
Crescimento
Espera-se que o número de vagas de emprego para 'Data Scientists' aumente 36,0% até 2033
As projeções atualizadas são devidas 09-2025.
Salários
Em 2023, o salário anual mediano para 'Data Scientists' foi de $ 108.020, ou $ 51 por hora
'Data Scientists' receberam 124,8% a mais do que o salário médio nacional, que era de $ 48.060
Volume
A partir de 2023 havia 192.710 pessoas empregadas como 'Data Scientists' dentro dos Estados Unidos.
Isso representa cerca de 0,13% da força de trabalho empregada em todo o país
Dito de outra maneira, cerca de 1 em 787 pessoas são empregadas como 'Data Scientists'.
Descrição do trabalho
Produza inteligência financeira e de mercado consultando repositórios de dados e gerando relatórios periódicos. Elabore métodos para identificar padrões e tendências de dados em fontes de informação disponíveis.
SOC Code: 15-2051.01
Comentários
Leave a comment
The bulk of my time as a BI analyst is spent on 2 things. The first is working with domain stakeholders without technical knowledge (marketers in my case). This can often be like herding cats. Lots of people will have lots of different opinions on what KPIs they want to track or how to track them. And often they don't really understand the data limitations of what we can and can't report on, so I need to be there to provide guidance. Much of my job is spent guiding these people along, often massaging their egos along the way, so that the wider group of people arrives at a consensus.
The second is ETL. Even with whole teams of data engineers and operations managers, data is very rarely centralised into a single and easy to understand model. I work with about half a dozen different types of data sources (from AWS to Google Sheets). Each of these have hundreds of different indexes and many of those indexes have hundreds of fields. A tiny fraction of these fields have any kind of documentation and so all you have to go on is the metadata and the name of the db managers who put it together. Actually tracking down the data you need requires getting really into the weeds and following up with multiple people to try to track down who actually knows where to find the data you're looking for. That's just nowhere near enough data for an AI to get a hold of the data it needs.
It is a field where people usually believe in other people rather than AI.
The ETL process is also a complicated, one which most AI is not nor ever may be able to handle, data needs to be cleaned and standardized before AI can take a crack at it, the "AI" and yes I have to put that in quotes does not understand the context of anything, it is a prediction model using gradient boosters that performs quite well under controlled circumstances, thrown into any critical thought role it starts to lose pace. Furthermore nobody who works in the AI space authoring models ands understands the inner workings of "AI" treats this as anything more than a highly sophisticated toy...maybe in another 10 years we can come back to this question and see if we should start to worry.
I spend half of my time maintaining, tweaking, and fixing automation jobs - these include dashboards, data sets, and database tables.
It requires a technical person who is also an expert in their business domain to translate business requirements into data or reports that others need.
Deixe uma resposta sobre esta ocupação