Business Intelligence Analisten
Werkgelegenheidsgegevens zijn specifiek voor deze beroepsgroep niet beschikbaar van het Bureau voor Arbeidsstatistieken, dus we gebruiken de gegevens van Data Scientists.
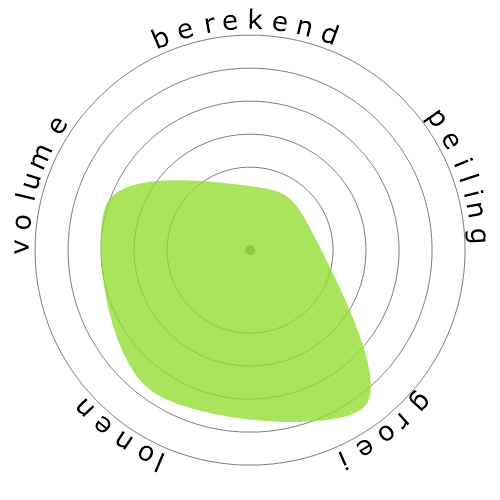



Wilt u deze samenvatting op uw site? Insluitcode:
Risico van automatisering
Hoge Risico (61-80%): Banen in deze categorie lopen een aanzienlijk risico door automatisering, aangezien veel van hun taken gemakkelijk kunnen worden geautomatiseerd met behulp van huidige of nabije toekomstige technologieën.
Meer informatie over wat deze score is en hoe deze wordt berekend, is beschikbaar hier.
Gebruikerspeiling
Onze bezoekers hebben gestemd dat ze niet zeker weten of dit beroep zal worden geautomatiseerd. De automatiseringsrisicograad die we echter hebben gegenereerd, suggereert een veel hogere kans op automatisering: 69% kans op automatisering.
Wat denk je dat het risico van automatisering is?
Wat is de kans dat Business Intelligence Analisten binnen de komende 20 jaar vervangen zal worden door robots of kunstmatige intelligentie?
Gevoel
De volgende grafiek(en) zijn opgenomen waar er een aanzienlijke hoeveelheid stemmen is om betekenisvolle gegevens te leveren. Deze visuele weergaven tonen de resultaten van gebruikerspolls in de loop van de tijd, en geven een belangrijke indicatie van sentimenttrends.
Gevoel over tijd (jaarlijks)
Groei
Het aantal 'Data Scientists' vacatures zal naar verwachting stijgen met 35,8% tegen 2032
Bijgewerkte prognoses zijn verschuldigd 09-2023.
Lonen
In 2022 was het mediane jaarloon voor 'Data Scientists' $ 103.500, of $ 49 per uur
'Data Scientists' werden 123,5% hoger betaald dan het nationale mediane loon, dat op $ 46.310 stond.
Volume
Vanaf 2022 waren er 159.630 mensen in dienst als 'Data Scientists' binnen de Verenigde Staten.
Dit vertegenwoordigt ongeveer 0,11% van de werkende bevolking in het hele land.
Anders gezegd, ongeveer 1 op de 926 mensen is werkzaam als 'Data Scientists'.
Functieomschrijving
Financiële en marktinformatie produceren door gegevensopslagplaatsen te bevragen en periodieke rapporten te genereren. Bedenk methoden voor het identificeren van gegevenspatronen en trends in beschikbare informatiebronnen.
SOC Code: 15-2051.01
Middelen
Als u overweegt een nieuwe carrière te beginnen, of van baan wilt veranderen, hebben we een handige zoektool voor banen gemaakt die u mogelijk helpt bij het vinden van die perfecte nieuwe functie.
Opmerkingen
The ETL process is also a complicated, one which most AI is not nor ever may be able to handle, data needs to be cleaned and standardized before AI can take a crack at it, the "AI" and yes I have to put that in quotes does not understand the context of anything, it is a prediction model using gradient boosters that performs quite well under controlled circumstances, thrown into any critical thought role it starts to lose pace. Furthermore nobody who works in the AI space authoring models ands understands the inner workings of "AI" treats this as anything more than a highly sophisticated toy...maybe in another 10 years we can come back to this question and see if we should start to worry.
I spend half of my time maintaining, tweaking, and fixing automation jobs - these include dashboards, data sets, and database tables.
It requires a technical person who is also an expert in their business domain to translate business requirements into data or reports that others need.
Laat een reactie achter over dit beroep